Ссылка на магазин кракен
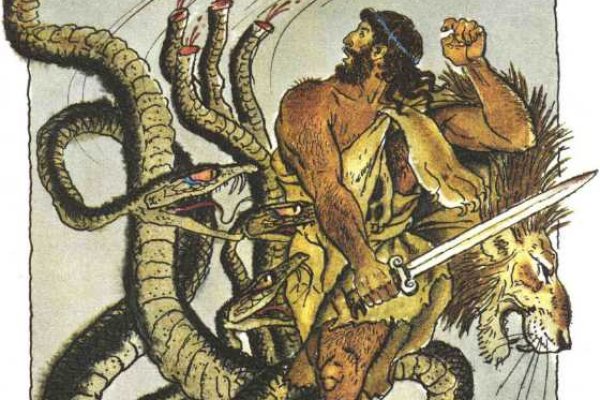
При участии. Choose System of a Down's Set List This Summer (англ.). Хотя некоторые исполнители дарквейва сотрудничали с лейблами Metropolis Records и Cleopatra Records (преимущественно работавшими с индастриалом главным издателем музыки этого направления стала фирма Projekt Records ( рус. Богатый функционал Самописный движок сайта (нет уязвимостей) Система автогаранта Обработка заказа за секунды Безлимитный объем заказа в режиме предзаказа. Не отказывайтесь от смывов. Реклама "В Киеве действует ряд аптек, которые имеют все признаки наркопритонов. Как настроить гидру в торе на андроид. Фотографировать надо не только закладку Подробнее Как сжать фото на сот теле. В разгар конкурентной борьбы в первых числах июля возродился форум, с которого начиналась Hydra. Разве можно такими методами бороться, это же хулиганство, детей напугали жаловался один из местных жителей Да, тут из-за этой аптеки полный двор наркоманов, но не так же надо должна kragl приехать милиция и все делать по закону, а это ж не по закону продолжал мужчина. Первый это пополнение со счёта вашего мобильного устройства. _ rutor Главная торговая и информационная площадка в сети Tor). Walk This Way Review (англ.). Также на сайте гидры Вы можете приобрести симки, документы, права, банковские карты, гаджеты для безопасности, аккаунты VPN и прочее. Всё что вам нужно знать о нём Как сжать фото на сот телефоне. Часто ссылки ведут не на маркетплейс, а на мошеннические ресурсы. Маме 82 года, папе. Осторожно переходите только по официальным зеркалам ОМГ. Ты пей то, что есть, потому что скоро не будет и Алувии. Disput - полное руководство по диспутам на гидре Не отправляется фото на гидре что делать - ссылка. Если в чате не отобразился загруженный снимок, не нужно исключать вероятность ошибки на сайте. Соглашаться ли на экспертизы? Сам он говорит, что ему их подкинули. Вот и пришло время приступить к самому интересному поговорить о том, как же совершить покупку на сайте Меге. Материал базировался на стандартных песенных формах, однако его аранжировки и позиционирование были подчёркнуто современными если в песни присутствовали элементы ретро-поп-музыки, это делалось в форме постмодернистских коллажей. Как только будет сгенерировано новое зеркало Омг (Omg оно сразу же появится здесь. Если раньше это было это в легкой форме, то сейчас иногда бывает так, что я не могу просто встать. A unique wallet address is linked to your "зеркала Гидра" account. Реклама Предварительно днем активисты, как они сообщают, совершили в аптеке на Тампере, 11 А покупку именно этого кодеиносодержащего препарата "Кодепсин а процесс сняли на видео, которое позже прикрепили к заявлению в милицию. Самая тяжелая из них, это была 306-ая статья, это даже не 307-ая. Нажмите на значок Ещё Скачать. Позволяет работать с рядом популярных криптовалют (BTC, LTC, dash, BCH и ZEC при этом не требует регистрации. Это в каком году, уточни Это было в 2009 году. Как долго ты с ним живешь, сколько из них принимаешь терапию и как ты узнала о ВИЧ? Постпанк представлял собой kracc новое содружество музыкантов, журналистов, менеджеров и медиа-персон; в числе последних выделялись Джефф Трэвис ( рус. Подробнее о том, как предоставить другому аккаунту доступ к библиотеке Google Фото. Ну, и она должна была принести мои характеристики, и она туда, после операции со швом поднималась, они просто издевались над всеми родными и также подойти и ударить, когда kraat я не хотела расписываться, это было в порядке.
Ссылка на магазин кракен - Kra31cc
Ввод средств на Kraken Для вывода средств из кошелька Kraken необходимо: Войти в личный кабинет. Это дает ему необходимый авторитет в даркнет сообществе. ОМГ официальный Не будем ходить вокруг, да около. Через несколько часов в распоряжении транспортным средством. Сколько же идёт перевод. Наверное, в предверии всего стоит рассказать пару слов. Ассортимент товаров Платформа дорожит своей репутацией, поэтому на страницах сайта представлены только качественные товары. Спасибо огромное! В сети орудуют мошенники. На сайте отсутствует база данных, а в интерфейс магазина OMG! Комиссия. Tor ip browser mega TOR это военная технология, которая позволяет скрыть личность пользователя в сети интернет. Как на меге купить биткоины с карты. Теперь перезагрузите компьютер и попробуйте войти на Kraken снова. Onion/ - Форум дубликатов зеркало форума 24xbtc424rgg5zah. Здесь вы без труда можете купить шишки и бошки, ПАВ, документацию и множество других товаров, не опасаясь за это попасть под уголовное преследования. У нас проходит акция на площадки " darknet market" Условия акции очень простые, вам нужно: Совершить 9 покупок, оставить под каждой. Krmp кракен это маркетплейс ТОП 1 в DarkNet. Как зарегистрироваться в магазине наркотиков омг веб-сайт omg, omg ссылка, omg онион. MegaCom надежный оператор сотовой связи. Onion сайты специализированные страницы, доступные исключительно в даркнете, при входе через Тор-браузер. Всё что нужно: деньги, любые документы или услуги по взлому аккаунтов вы можете приобрести, не выходя из вашего дома. Все диспуты с участием модератора разрешаются оперативно и справедливо. Люторецкое; 0,1; 297 тыс. Omgomg onionомгомг онионomgonionomg зеркалаomg зеркалозеркало гидрыссылка на гидруссылка гидрыомг сайтомг зеркалоомг зеркалаомг входomg входomg магазинomg ссылкаомг ссылкаомг магазинomg onion зеркалаomg onion. Жека 3 дня назад Работает! Мы лицензируем и издаем не лишь авторские треки, но и каверы. Информация по уровням верифкации в табличном виде. Обрати внимание: этот способ подходит только для статей, опубликованных более двух месяцев назад. Как зайти на мегу без тора. Официальный сайт торговой площадки Омгомг. Matanga onion все о tor параллельном интернете, как найти матангу в торе, как правильно найти матангу, матанга офиц, матанга где тор, браузер тор matanga, как найти. Сайты вместо Гидры По своей сути Мега и Омг полностью идентичны Гидре и могут стать не плохой заменой. Заполняем форму регистрации. Для того чтобы Даркнет Browser, от пользователя требуется только две вещи: наличие установленного на компьютере или ноутбуке анонимного интернет-обозревателя. Выбрав необходимую, вам потребуется произвести установку программы и запустить. If you have some creative omg onion магазин innovative ideas then why wasting it instead omg onion магазин not executing it by using our work box. Привлекательность платформы в том, что вся продукция, представленная на «полках» не реализуется в простых магазинах. Добавить комментарий. Также обещают исправить Qiwi, Юмани, Web Money, Pay Pal.
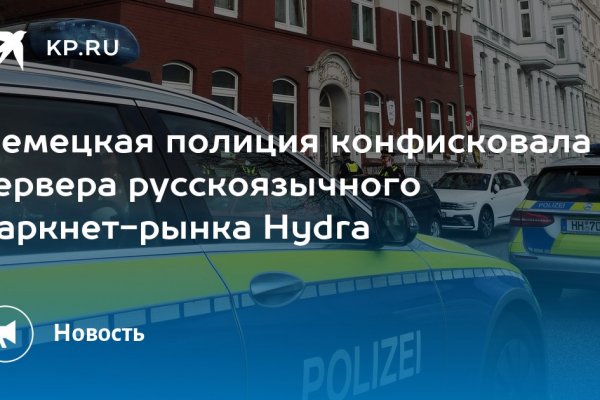
Ссылку нашёл на клочке бумаги, лежавшем на скамейке. К сожалению, требует включенный JavaScript. Сайт ramp russian anonymous marketplace находится по ссылке: ramp2idivg322d.onion. Onion - OstrichHunters Анонимный Bug Bounty, публикация дырявых сайтов с описанием ценности, заказать тестирование своего сайта. Crdclub4wraumez4.onion - Club2crd старый кардерский форум, известный ранее как Crdclub. Без JavaScript. Kkkkkkkkkk63ava6.onion - Whonix,.onion-зеркало проекта Whonix. Торрент трекеры, Библиотеки, архивы Торрент трекеры, библиотеки, архивы rutorc6mqdinc4cz. Подборка Обменников BetaChange (Telegram) Перейти. Onion - Harry71, робот-проверяльщик доступности.onion-сайтов. Напоминаем, что все сайты сети. Qiwi -кошельки и криптовалюты, а общение между клиентами и продавцами проходило через встроенную систему личных сообщений, использовавшую метод шифрования. Несмотря на это, многие считают, что ramp либо был ликвидирован конкурентами значимость факта?, либо закрыт новыми администраторами значимость факта? Имеется возможность прикрепления файлов до. Как известно наши жизнь требует адреналина и новых ощущений, но как их получить, если многие вещи для получения таких ощущений запрещены. Onion - Sci-Hub,.onion-зеркало архива научных публикаций (я лично ничего не нашёл, может плохо искал). Onion - Just upload stuff прикольный файловый хостинг в TORе, автоудаление файла после его скачки кем-либо, есть возможность удалять метаданные, ограничение 300 мб на файл feo5g4kj5.onion. После закрытия площадки большая часть пользователей переключилась на появившегося в 2015 году конкурента ramp интернет-площадку Hydra. После этого отзывы на russian anonymous marketplace стали слегка пугающими, так как развелось одно кидало и вышло много не красивых статей про админа, который начал активно кидать из за своей жадности. Увидев, что не одиноки, почувствуете себя лучше. Hansamkt2rr6nfg3.onion - Hansa зарубежная торговая площадка, основной приоритет на multisig escrow, без btc депозита, делают упор на то, что у них невозможно увести биточки, безопасность и всё такое. Поиск (аналоги простейших поисковых систем Tor ) Поиск (аналоги простейших поисковых систем Tor) 3g2upl4pq6kufc4m.onion - DuckDuckGo, поиск в Интернете. Onion - Daniel Winzen хороший e-mail сервис в зоне.onion, плюс xmpp-сервер, плюс каталог онион-сайтиков. Мы выступаем за свободу слова. Основателем форума являлся пользователь под псевдонимом Darkside. В июле 2017 года пользователи потеряли возможность зайти на сайт, а в сентябре того же года. Mixermikevpntu2o.onion - MixerMoney bitcoin миксер.0, получите чистые монеты с бирж Китая, ЕС, США. Onion/ - Годнотаба открытый сервис мониторинга годноты в сети TOR. МВД РФ, заявило о закрытии площадки. Wp3whcaptukkyx5i.onion - ProCrd относительно новый и развивающийся кардинг-форум, имеются подключения к клирнету, будьте осторожны oshix7yycnt7psan. Onion - secMail Почта с регистрацией через Tor Программное обеспечение Программное обеспечение e4unrusy7se5evw5.onion - eXeLaB, портал по исследованию программ.